25 July 2019
The Art of Statistics:
Learning from Data
David Spiegelhalter
2019, Pelican, 448 pages,
ISBN 9780241398630
Reviewer: Bridget Rosewell, Volterra Partners
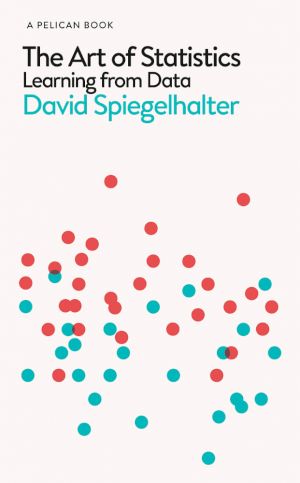
You may think that you already know everything likely to be in this book. You’ve done a statistics course, and one in econometrics and you’re up to speed on R and Python. Nonetheless, you should read this book. More, you should give it to your clients and colleagues. Speigelhalter is an expert communicator: a regular contributor to Tim Harford’s “ More or Less” on Radio 4, for example. It is not just the statistical knowledge that he imparts, but also the techniques and pitfalls in reaching conclusions and getting them over to the audience. These are areas where economists need to improve.
The first three chapters are about data, data visualisation and communication. They stress the need for good sense checking and the need for making sure the audience understand absolute risk as well as relative. He recommends never using odds ratios which your average policy maker will misunderstand. These are good crib sheets.
From there, we are into the challenges of causation. I was going to write about the more meaty challenges, but that devalues the really important questions of choosing, assessing and visualising the data. Garbage in is garbage out and we do often forget this. Worse, we sometimes believe that any data is better than no data. I don’t think this is true. Wrong data can lead to apparently plausible conclusions which are misleading and drive wrong actions. No data is more likely to lead to caution and asking what outcomes a decision needs to be robust to, rather than assuming we can tell what the outcomes will be.
Three chapters look at regression, algorithms, prediction and uncertainty. There’s a concise explanation of random forest, support vectors, neural networks and k nearest neighbours, with which you may be less familiar. He applies these to an example of the chances of surviving the Titanic disaster, which brings them neatly to life and also displays their weaknesses of overfitting, black box outcomes and possible data set biases. Finally he looks at bootstrapping, reliability of parameters and the need to report uncertainty intervals. These chapters should not only help you decide on the appropriate techniques that you might want to use on your data but also give you some cautionary tales to beware. Users of economists’ services love point estimates. Some years ago, I tried to develop a forecasting service which would have ranges and would ask the client what range they wanted to be robust to in their business plan. I was naïve. No one wanted such a service. They wanted a number to plug into their business plan spreadsheet, which itself came up with a point estimate. Our biggest challenge as analysts is to get users to accept ranges rather than point estimates, whether we are looking at GDP or the cost of HS2.
So how sure are you? Putting probability and statistics together is where we can come up with conclusions about what matters. At the heart of probably every piece of advice that a professional economist gives is a statistical inference. What is the likelihood that your sample is really representative, and what judgement must you bring to bear to deal with non-random causes that are not in your data but might be important. Probability may work over large samples but at best it will only give you the chance of rain. A rare event happening today does not rule it out happening tomorrow. Spiegelhalter argues that probability is against intuition and is a hard idea. Casual empiricism in our own lives will bear this out.
After all this, hypothesis testing raises the level of difficulty, or perhaps the challenge to our usual thinking, in showing how p-values and null hypothesis testing can lead you astray. Specifically he sets out the conclusion that a p-value does not measure the probability that a hypothesis is true. Frankly I’m still struggling to get this sufficiently clear in my head so that I will always recognise the prosecutor’s fallacy. This is the difference between, in Spiegelhalter’s example, the proposition that only 10% of women without breast cancer would get a positive mammogram (supported by data) and that which says only 10% of women with a positive mammogram do not have breast cancer (not in the data). It’s worth looking up the American Statistical Association’s 6 tests, but here they are anyway:
The statement’s six principles, many of which address misconceptions and misuse of the p-value, are the following:
1. P-values can indicate how incompatible the data are with a specified statistical model.
2. P-values do not measure the probability that the studied hypothesis is true, or the probability that the data were produced by random chance alone.
3. Scientific conclusions and business or policy decisions should not be based only on whether a p-value passes a specific threshold.
4. Proper inference requires full reporting and transparency.
5. A p-value, or statistical significance, does not measure the size of an effect or the importance of a result.
6. By itself, a p-value does not provide a good measure of evidence regarding a model or hypothesis.
Enjoy!
I was pleased that after this, Bayesian inference gets a look in. The idea that we should incorporate prior postulates into inference sounds like common sense in some ways, and almost certainly lies behind hypothesis formulation and data collection choices. So beware saying that it is irrelevant. Modelling strategies that incorporate feedback loops in their construction are generally thinking in Bayesian terms as parameters are updated based on experience. And of course there is no null hypothesis to test, nor an uncertainty about the prosecutor’s fallacy as the posterior calculation of probabilities generates a confidence interval about the true model bringing together both the prior likelihood and the data.
Finally, Spiegelhalter tackles the reproducibility crisis, over-egging of results and questionable research practices. He offers a call to arms to ensure that our analyses are properly planned to answer specific questions, rest on reliable data, provide ranges of error, and clear assumptions. These all sound obvious and indeed were also some of the recommendations in a Government Office for Science Blackett review of which I was a co-author: https://www.gov.uk/government/publications/computational-modelling-blackett-review.
Having agreed recommendations and ways of working is, however, easier to say than to do. The SPE has a particular responsibility for fostering good ways of working and indeed getting them better understood. Good books which set this out clearly should be part of the armoury. This is one such.